This report examines the surging healthcare costs in the U.S. from 1980 to 2014, revealing the factors behind its status as one of the world’s most expensive countries for healthcare.
Author
Brian Cervantes Alvarez
Published
December 12, 2022
Modified
June 16, 2025
Yapper Labs | AI Summary
Model: ChatGPT 4.5
I conducted a comprehensive statistical analysis of U.S. healthcare spending from 1980 to 2014, performing extensive data wrangling, visualization, and regional comparisons to uncover expenditure trends. My analysis revealed healthcare costs increased by over 500% nationally, with Personal Healthcare expenses alone rising from approximately $10,000 to nearly $80,000 per capita. Notably, significant regional disparities were identified, with spending in traditionally cheaper regions like New England, Plains, and Rocky Mountains still growing by 18%, highlighting persistent nationwide increases.
Abstract
Since 1980, healthcare costs in the United States have been consistently increasing across all categories. Various factors contribute to this rise, such as population growth and higher wages for doctors. This report examines expenditure trends from 1980 to 2005, extending up to 2014. The findings reveal an unprecedented surge in healthcare costs across every sector in the United States. Consequently, this report sheds light on the reasons behind the country’s reputation as one of the world’s most expensive nations in terms of healthcare.
Introduction
Healthcare plays a crucial role in our lives, providing essential support for our well-being and longevity. However, healthcare spending continues to soar annually. This report uncovers the alarming reality of escalating healthcare expenditure, presenting a visual representation of each component. It explores overall national spending and delves into individual categories, demonstrating the persistent upward trend in healthcare costs.
Background
The dataset utilized for this report is titled “US Healthcare Spending Per Capita” and was obtained from Kaggle. The dataset’s format posed a challenge, as it followed a wide format with numerous columns and few rows. Notably, the years were presented in the format “Y####,” initially impeding analysis. However, by employing pivoting techniques and manipulating the strings, the dataset was transformed, enabling comprehensive analysis. The subsequent section outlines the complete step-by-step process.
Methodology
To begin, it is essential to assess whether the data is in a “wide” or “long” format. This involves examining the number of rows and columns to facilitate necessary data wrangling.
Version Control
Make sure to use RStudio’s version 2023.12.1 or higher
Earlier, we noticed that the dataset had a wide format, which means the years were in separate columns. To make it easier to analyze, we rearranged the data using a special technique. We combined the year columns into a single “Year” column and placed their corresponding values in a new column called “Cost.”
We also made some adjustments to the “Year” column by removing a specific symbol and converting it to numbers. This way, we can work with the years as numeric values instead of text.
Additionally, we transformed certain columns into categories, which help us group and analyze the data more effectively. These categories include “Item,” “Region_Name,” “Group,” and “State_Name.”
Finally, we selected specific columns, including “Item,” “Region_Name,” “State_Name,” “Year,” and “Cost,” to focus on for further analysis. This will provide us with a clearer understanding of the data.
# A tibble: 6 × 5
Item Region_Name State_Name Year Cost
<fct> <fct> <fct> <dbl> <dbl>
1 Personal Health Care United States <NA> 1980 216977
2 Personal Health Care United States <NA> 1981 251789
3 Personal Health Care United States <NA> 1982 283073
4 Personal Health Care United States <NA> 1983 311677
5 Personal Health Care United States <NA> 1984 341645
6 Personal Health Care United States <NA> 1985 376376
Rising Health Care Costs
Let’s jump right into the first visualization. It’s evident that healthcare spending has been consistently increasing and shows no signs of slowing down. This graph focuses on the years 1980 to 2005, highlighting the era of escalating healthcare costs.
Dominant Spending Categories: Personal, Hospital, and Physician & Clinical Care
Wow! Personal health care expenses skyrocketed from around $10K to nearly $80K within a relatively short period. Both Hospital and Clinical Care play significant roles in healthcare spending. Surprisingly, all three categories follow a similar upward trend, which reveals some unsettling information.
Consistent Trends Across Regions
It’s disheartening to report that every region has been experiencing relentless growth in healthcare spending. The trend lines for each region are strikingly similar and proportionate to one another. Notably, the Mideast stands out as the most expensive region, while the Rocky Mountains region appears to have comparatively lower healthcare costs. It’s important to note that this analysis considers spending up to 2005, and we can hope for potential changes by 2014.
Persistent Trends: Rising Healthcare Spending Across U.S. Regions
The bar chart vividly demonstrates the ongoing trends in healthcare spending across different regions. Notably, the Plains, New England, and the Rocky Mountains regions emerge as some of the lowest in terms of medical funding. Surprisingly, their costs can be as low as one-third compared to the most expensive regions. This stark contrast highlights the significant disparities in healthcare expenditure throughout the United States.
Far West: A Surprising 3rd Place in Healthcare Spending
In 2014, the Far West region experienced a significant surge in healthcare spending, landing them in the 3rd position. This unexpected leap challenges the assumption that states within this region are heavy spenders. However, the subsequent graphic reveals an intriguing revelation that contradicts this perception.
[1] "$21.81M"
Oregon: 3rd Place, but Don’t Be Deceived!
Surprisingly, Oregon ranks 3rd in healthcare spending. However, let’s not overlook the undeniable fact that California claims the top spot. The massive population size of California is a significant contributing factor to its high expenditure. Although this report doesn’t delve into the specific reasons, it’s plausible that further analysis would align the Far West region more closely with the spending patterns observed in the Plains or New England regions.
Inappropriate Model: Linear Fit Inadequate for the Data
At first glance, the model may seem impressive with an adjusted R-squared value of 0.8572. However, this is deceptive. It’s crucial to note that this model is highly inaccurate and strongly discouraged. The analysis reveals no correlation between Cost and Region_Name per Year, a finding consistent with the filtered dataset covering the years 1980 to 2014.
The residual plots provide clear evidence against a linear fit. The Residuals vs Fitted plot indicates a clear quadratic relationship rather than a linear one. The Q-Q plot deviates from linearity, exhibiting multiple curves along the fitted line. Additionally, the scale-location plot highlights that this model is fundamentally unsuitable for the data.
It is evident that a linear fit is not the appropriate choice for accurately modeling this dataset.
Df Sum Sq Mean Sq F value Pr(>F)
Region_Name 7 1.185e+09 1.693e+08 95.9 <2e-16 ***
Year 1 1.391e+09 1.391e+09 787.9 <2e-16 ***
Residuals 199 3.514e+08 1.766e+06
---
Signif. codes: 0 '***' 0.001 '**' 0.01 '*' 0.05 '.' 0.1 ' ' 1
Significant Differences in Means among Regions
My objective was to investigate whether there were significant differences in the means of each region’s spending over the years. To start, I utilized the LeveneTest to examine the importance of variance (i.e., the spread of spending) across regions. Both tests yielded remarkably small p-values, indicating that three regions had substantially different variances compared to the others.
Building on this, I employed the TukeyHsd test to confirm if these differing variances were reflected in the means. As anticipated from the LeveneTest results, the means of these regions indeed exhibited significant differences. Notably, New England, Plains, and Rocky Mountains had considerably lower average spending. However, despite their comparatively lower spending, these regions still followed the overall growth trend, with an increase of 18% since 2005.
[1] "The Average Spending In The Expensive Regions since 2005 = $5333.29"
[1] "The Average Spending In The Expensive Regions since 2014= $7724.45"
The cost of healthcare in the United States has increased more than fivefold between 1980 and 2014. Across regions, there is a consistent upward trend in healthcare spending with no clear indications of a decrease. Although some regions are less expensive than others, their growth rates align with the national average. Personal health care spending, which averaged around $10,000 in 1980, has significantly risen to nearly $80,000 in 2014.
As of 2014, the Mideast, Great Lakes, and Far West regions rank as the top three most expensive regions, while the Rocky Mountains, New England, and Plains regions are the least expensive.
Within the Far West region, Oregon stands out as the third most expensive state.
Conclusion
The United States continues to experience escalating healthcare expenditures, raising concerns about the affordability of personal health care. The substantial increase of approximately $70,000 over a span of 35 years far exceeds inflation expectations. It would have been beneficial to have inflation-adjusted values in the dataset, allowing for a more comprehensive analysis and deeper insights.
Further exploration can be done to investigate potential statistical significance between individual states and their spending patterns. This avenue remains open for future researchers to delve into for a more in-depth understanding of healthcare expenditure variations.
Data References
Fox, John, and Sanford Weisberg. 2019. An R Companion to Applied Regression. Third. Thousand Oaks CA: Sage. https://www.john-fox.ca/Companion/.
Grolemund, Garrett, and Hadley Wickham. 2011. “Dates and Times Made Easy with lubridate.”Journal of Statistical Software 40 (3): 1–25. https://www.jstatsoft.org/v40/i03/.
Kim, Albert Y., Chester Ismay, and Max Kuhn. 2021. “Take a Moderndive into Introductory Linear Regression with r.”The Journal of Open Source Education 4 (41, 115). https://doi.org/10.21105/jose.00115.
Schloerke, Barret, Di Cook, Joseph Larmarange, Francois Briatte, Moritz Marbach, Edwin Thoen, Amos Elberg, and Jason Crowley. 2024. GGally: Extension to Ggplot2. https://ggobi.github.io/ggally/.
Spinu, Vitalie, Garrett Grolemund, and Hadley Wickham. 2024. Lubridate: Make Dealing with Dates a Little Easier. https://lubridate.tidyverse.org.
Waring, Elin, Michael Quinn, Amelia McNamara, Eduardo Arino de la Rubia, Hao Zhu, and Shannon Ellis. 2022. Skimr: Compact and Flexible Summaries of Data. https://docs.ropensci.org/skimr/.
Wickham, Hadley. 2016. Ggplot2: Elegant Graphics for Data Analysis. Springer-Verlag New York. https://ggplot2.tidyverse.org.
Wickham, Hadley, Mara Averick, Jennifer Bryan, Winston Chang, Lucy D’Agostino McGowan, Romain François, Garrett Grolemund, et al. 2019. “Welcome to the tidyverse.”Journal of Open Source Software 4 (43): 1686. https://doi.org/10.21105/joss.01686.
Wickham, Hadley, Winston Chang, Lionel Henry, Thomas Lin Pedersen, Kohske Takahashi, Claus Wilke, Kara Woo, Hiroaki Yutani, Dewey Dunnington, and Teun van den Brand. 2024. Ggplot2: Create Elegant Data Visualisations Using the Grammar of Graphics. https://ggplot2.tidyverse.org.
Wickham, Hadley, Romain François, Lionel Henry, Kirill Müller, and Davis Vaughan. 2023. Dplyr: A Grammar of Data Manipulation. https://dplyr.tidyverse.org.
Wickham, Hadley, Jim Hester, and Jennifer Bryan. 2024. Readr: Read Rectangular Text Data. https://readr.tidyverse.org.
Wickham, Hadley, Thomas Lin Pedersen, and Dana Seidel. 2023. Scales: Scale Functions for Visualization. https://scales.r-lib.org.
Wickham, Hadley, Davis Vaughan, and Maximilian Girlich. 2024. Tidyr: Tidy Messy Data. https://tidyr.tidyverse.org.
Xie, Yihui. 2014. “Knitr: A Comprehensive Tool for Reproducible Research in R.” In Implementing Reproducible Computational Research, edited by Victoria Stodden, Friedrich Leisch, and Roger D. Peng. Chapman; Hall/CRC.
———. 2015. Dynamic Documents with R and Knitr. 2nd ed. Boca Raton, Florida: Chapman; Hall/CRC. https://yihui.org/knitr/.
———. 2024. Knitr: A General-Purpose Package for Dynamic Report Generation in r. https://yihui.org/knitr/.
This website uses Google Analytics to improve its functionality.
Yappify Chat Assistant
Yappify 1.0
RAG
Qwen 2.5
LLM
GPT-4
Coming Soon
Claude
Coming Soon
New Chat
Start a fresh conversation
Export Chat
Download conversation
JSON
JSON Format
Structured data for developers
XLSX
Excel Format
Spreadsheet with formatting
CSV
CSV Format
Simple comma-separated data
Search Website
Coming Soon
Yappify can make mistakes. Please double-check responses.
Source Code
---title: "Healthcare Spending Analysis" author: "Brian Cervantes Alvarez"date: "12-12-2022"date-modified: todaydescription: "This report examines the surging healthcare costs in the U.S. from 1980 to 2014, revealing the factors behind its status as one of the world's most expensive countries for healthcare."teaser: "In-depth exploration of rising U.S. healthcare costs from 1980 to 2014."image: /assets/images/healthcareSpending.jpegcategories: ["Statistics"]bibliography: "bibliography.bib"nocite: | @*format: html: code-fold: true code-tools: true page-layout: article toc: true toc-location: right html-math-method: katexexecute: message: false warning: false echo: falseai-summary: banner-title: "Yapper Labs | AI Summary" model-title: "Model: ChatGPT 4.5" model-img: "/assets/images/OpenAI-white-monoblossom.svg" summary: "I conducted a comprehensive statistical analysis of U.S. healthcare spending from 1980 to 2014, performing extensive data wrangling, visualization, and regional comparisons to uncover expenditure trends. My analysis revealed healthcare costs increased by over 500% nationally, with Personal Healthcare expenses alone rising from approximately $10,000 to nearly $80,000 per capita. Notably, significant regional disparities were identified, with spending in traditionally cheaper regions like New England, Plains, and Rocky Mountains still growing by 18%, highlighting persistent nationwide increases."---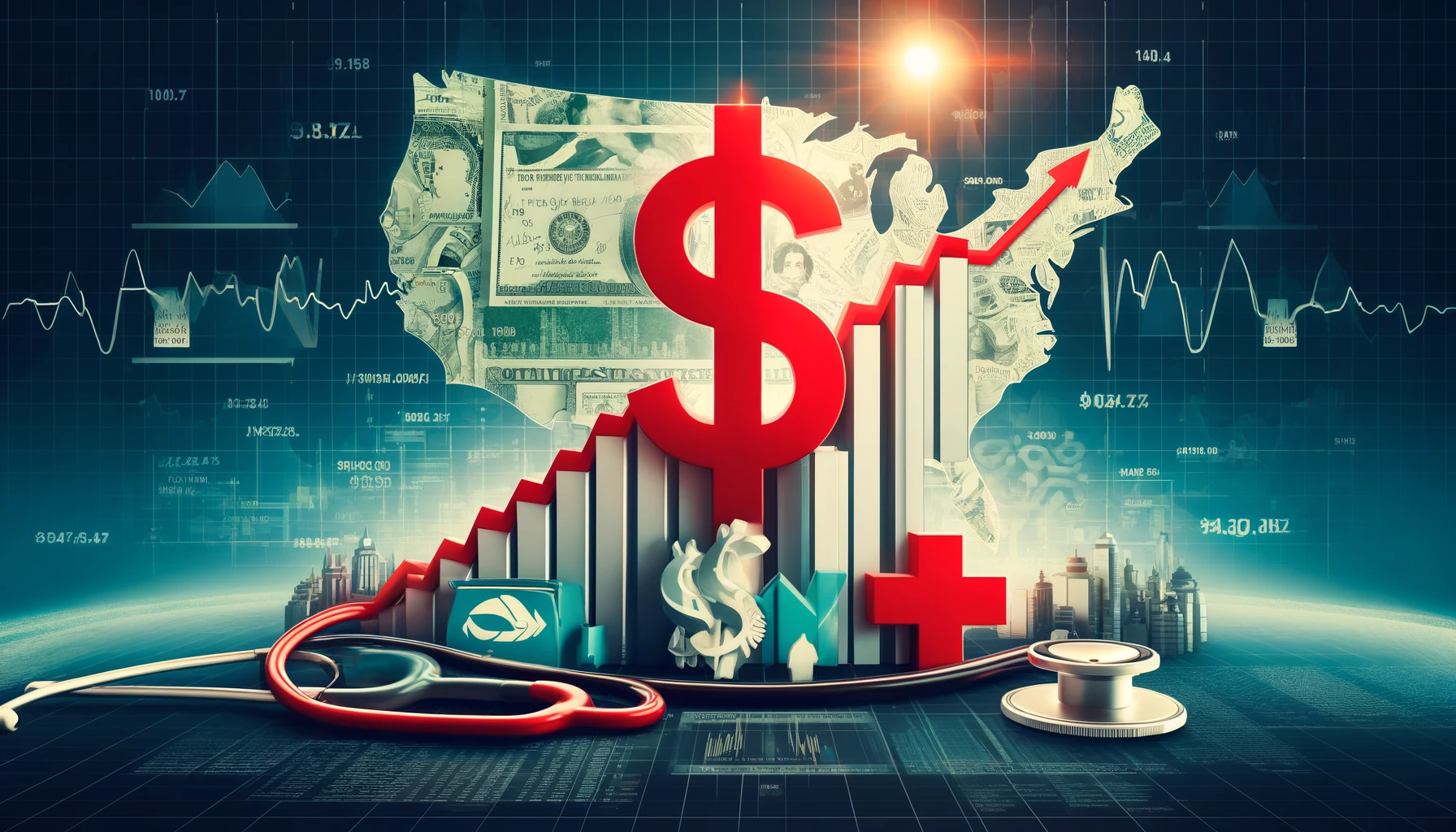## AbstractSince 1980, healthcare costs in the United States have been consistently increasing across all categories. Various factors contribute to this rise, such as population growth and higher wages for doctors. This report examines expenditure trends from 1980 to 2005, extending up to 2014. The findings reveal an unprecedented surge in healthcare costs across every sector in the United States. Consequently, this report sheds light on the reasons behind the country's reputation as one of the world's most expensive nations in terms of healthcare.## IntroductionHealthcare plays a crucial role in our lives, providing essential support for our well-being and longevity. However, healthcare spending continues to soar annually. This report uncovers the alarming reality of escalating healthcare expenditure, presenting a visual representation of each component. It explores overall national spending and delves into individual categories, demonstrating the persistent upward trend in healthcare costs.## BackgroundThe dataset utilized for this report is titled "US Healthcare Spending Per Capita" and was obtained from Kaggle. The dataset's format posed a challenge, as it followed a wide format with numerous columns and few rows. Notably, the years were presented in the format "Y####," initially impeding analysis. However, by employing pivoting techniques and manipulating the strings, the dataset was transformed, enabling comprehensive analysis. The subsequent section outlines the complete step-by-step process.## MethodologyTo begin, it is essential to assess whether the data is in a "wide" or "long" format. This involves examining the number of rows and columns to facilitate necessary data wrangling.::: {.callout-warning}## Version ControlMake sure to use RStudio's version 2023.12.1 or higher:::```{r}library(tidyverse)library(skimr)library(car)library(moderndive)library(knitr)library(GGally)library(scales)library(RColorBrewer)``````{r}ds <-read_csv("../../../assets/datasets/healthcareSpending.csv")head(ds, 5)names(ds)```Earlier, we noticed that the dataset had a wide format, which means the years were in separate columns. To make it easier to analyze, we rearranged the data using a special technique. We combined the year columns into a single "Year" column and placed their corresponding values in a new column called "Cost."We also made some adjustments to the "Year" column by removing a specific symbol and converting it to numbers. This way, we can work with the years as numeric values instead of text.Additionally, we transformed certain columns into categories, which help us group and analyze the data more effectively. These categories include "Item," "Region_Name," "Group," and "State_Name."Finally, we selected specific columns, including "Item," "Region_Name," "State_Name," "Year," and "Cost," to focus on for further analysis. This will provide us with a clearer understanding of the data.```{r message=FALSE, warning=FALSE, echo = FALSE}# Pivot Y{Year} columns into it's own Year columnds_years <- ds %>% pivot_longer( !(c( "Code", "Item", "Group", "Region_Number", "Region_Name", "State_Name", "Average_Annual_Percent_Growth" )), names_to = "Year", values_to = "Cost")# Get rid of "Y" symbol and make it numericds_years$Year <- as.numeric(gsub("Y", "", ds_years$Year))ds_years$Item <- gsub(" \\(Millions of Dollars\\)", "", ds_years$Item)ds_years$Item <- factor(ds_years$Item)ds_years$Region_Name <- factor(ds_years$Region_Name)ds_years$Group <- factor(ds_years$Group)ds_years$State_Name <- factor(ds_years$State_Name)ds_years <- ds_years %>% dplyr::select(Item, Region_Name, State_Name, Year, Cost)head(ds_years)```## Rising Health Care CostsLet's jump right into the first visualization. It's evident that healthcare spending has been consistently increasing and shows no signs of slowing down. This graph focuses on the years 1980 to 2005, highlighting the era of escalating healthcare costs.```{r message=FALSE, warning=FALSE, echo = FALSE}# Looking at overall U.S. healthcare costs since 2005usHealthCareSince2005 <- ds_years %>% filter((Year >= 1980 & Year <= 2005)) %>% group_by(Year) %>% summarise(Cost = mean(Cost))usHealthCareSince2005 %>% ggplot(aes(x = Year, y = Cost)) + geom_line(size = 1.2, color = "#b32134") + labs( x = "Year", y = "Cost (USD)", title = "U.S. Healthcare Spending Per Capita Since 2005", subtitle = "For All Healthcare Categories" ) + scale_y_continuous(labels = label_number(prefix = "$", suffix = "M", scale = 1e-3)) + theme_minimal()```### Dominant Spending Categories: Personal, Hospital, and Physician & Clinical CareWow! Personal health care expenses skyrocketed from around \$10K to nearly \$80K within a relatively short period. Both Hospital and Clinical Care play significant roles in healthcare spending. Surprisingly, all three categories follow a similar upward trend, which reveals some unsettling information.```{r message=FALSE, warning=FALSE, echo = FALSE}# Now looking at per category spendingusHealthCareSince2005 <- ds_years %>% filter((Year >= 1980 & Year <= 2005)) %>% arrange(Cost) %>% group_by(Item, Year) %>% summarise(Cost = mean(Cost))usHealthCareSince2005$Item <- factor(usHealthCareSince2005$Item, levels = c( "Personal Health Care", "Hospital Care", "Physician & Clinical Services", "Prescription Drugs", "Nursing Home Care", "Other Health, Residential, and Personal Care", "Dental Services", "Home Health Care", "Other Professional Services", "Other Non-durable Medical Products", "Durable Medical Products" ))usHealthCareSince2005 %>% ggplot(aes(x = Year, y = Cost)) + geom_line(color = "#b32134") + facet_wrap(~Item, scales = "free_y") + labs( x = "Year", y = "Cost (USD)", title = "U.S. Healthcare Spending Per Category Since 2005", subtitle = "Per Capita" ) + scale_y_continuous(labels = label_number(prefix = "$", suffix = "M", scale = 1e-3, big.mark = ",")) + theme_minimal() + theme( axis.text.x = element_text(angle = 90), strip.text = element_text(size = 4.2, face = "bold"), title = element_text(size = 10, face = "bold") )```### Consistent Trends Across RegionsIt's disheartening to report that every region has been experiencing relentless growth in healthcare spending. The trend lines for each region are strikingly similar and proportionate to one another. Notably, the Mideast stands out as the most expensive region, while the Rocky Mountains region appears to have comparatively lower healthcare costs. It's important to note that this analysis considers spending up to 2005, and we can hope for potential changes by 2014.```{r message=FALSE, warning=FALSE, echo = FALSE}regionHealthCareSince2005 <- ds_years %>% filter((Year >= 1980 & Year <= 2005) & Region_Name != "United States") %>% arrange(Cost) %>% group_by(Region_Name, Year) %>% summarise(Cost = mean(Cost))regionHealthCareSince2005 %>% ggplot(aes(x = Year, y = Cost)) + geom_line(color = "#b32134") + facet_wrap(~Region_Name, scales = "free_y") + labs( x = "Year", y = "Cost (USD)", title = "U.S. Healthcare Spending Per Region Since 2005" ) + scale_y_continuous(labels = label_number(prefix = "$", suffix = "M", scale = 1e-3, big.mark = ",")) + theme_minimal() + theme( axis.text.x = element_text(angle = 90), strip.text = element_text(size = 8, face = "bold"), title = element_text(size = 10, face = "bold") )```### Persistent Trends: Rising Healthcare Spending Across U.S. RegionsThe bar chart vividly demonstrates the ongoing trends in healthcare spending across different regions. Notably, the Plains, New England, and the Rocky Mountains regions emerge as some of the lowest in terms of medical funding. Surprisingly, their costs can be as low as one-third compared to the most expensive regions. This stark contrast highlights the significant disparities in healthcare expenditure throughout the United States.```{r message=FALSE, warning=FALSE, echo = FALSE}regionHealthCare2014 <- ds_years %>% filter((Year == 2014) & Region_Name != "United States") %>% arrange(Cost) %>% group_by(Region_Name) %>% summarise(Cost = mean(Cost))regionHealthCare2014 %>% ggplot(aes(x = reorder(Region_Name, Cost), y = Cost, fill = reorder(Region_Name, Cost))) + geom_col(color = "grey", show.legend = FALSE) + coord_flip() + labs( x = "Region", y = "Cost (USD)", title = "U.S. Healthcare Spending Per Region For 2014" ) + scale_fill_manual(values = c("#fff5f0", "#fee0d2", "#fcbba1", "#fc9272", "#fb6a4a", "#ef3b2c", "#cb181d", "#99000d")) + scale_y_continuous(labels = label_number(prefix = "$", suffix = "M", scale = 1e-3, big.mark = ",")) + theme_minimal() + theme(title = element_text(size = 10, face = "bold"))```### Far West: A Surprising 3rd Place in Healthcare SpendingIn 2014, the Far West region experienced a significant surge in healthcare spending, landing them in the 3rd position. This unexpected leap challenges the assumption that states within this region are heavy spenders. However, the subsequent graphic reveals an intriguing revelation that contradicts this perception.```{r message=FALSE, warning=FALSE, echo = FALSE}farWestHealthCare2014 <- ds_years %>% filter((Year == 2014) & Region_Name == "Far West") %>% arrange(Cost) %>% group_by(Region_Name) %>% summarise(Cost = mean(Cost))regionHealthCare2014 %>% ggplot(aes(x = reorder(Region_Name, Cost), y = Cost, fill = reorder(Region_Name, Cost))) + geom_col(color = "grey", show.legend = FALSE) + geom_col( data = farWestHealthCare2014, mapping = aes(x = reorder(Region_Name, Cost), y = Cost, fill = reorder(Region_Name, Cost)), color = "black", size = 1.2, show.legend = FALSE ) + geom_label( data = farWestHealthCare2014, mapping = aes( x = reorder(Region_Name, Cost), y = Cost, label = print(paste0("$", round(Cost / 1000, 2), "M")) ), size = 3, fill = "white", color = "black", hjust = 1.1, show.legend = FALSE ) + coord_flip() + labs( x = "Region", y = "Cost (USD)", title = "Far West Healthcare Spending For 2014" ) + scale_fill_manual(values = c("#fff5f0", "#fee0d2", "#fcbba1", "#fc9272", "#fb6a4a", "#ef3b2c", "#cb181d", "#99000d")) + scale_y_continuous(labels = label_number(prefix = "$", suffix = "M", scale = 1e-3, big.mark = ",")) + theme_minimal() + theme(title = element_text(size = 10, face = "bold"))```### Oregon: 3rd Place, but Don't Be Deceived!Surprisingly, Oregon ranks 3rd in healthcare spending. However, let's not overlook the undeniable fact that California claims the top spot. The massive population size of California is a significant contributing factor to its high expenditure. Although this report doesn't delve into the specific reasons, it's plausible that further analysis would align the Far West region more closely with the spending patterns observed in the Plains or New England regions.```{r message=FALSE, warning=FALSE, echo = FALSE}farWestStatesHealthCare2014 <- ds_years %>% filter((Year == 2014) & (Region_Name == "Far West") & (State_Name != "NA")) %>% arrange(Cost) %>% group_by(State_Name, Year) %>% summarise(Cost = mean(Cost))oregonHealthCare2014 <- ds_years %>% filter((Year == 2014) & (Region_Name == "Far West") & (State_Name == "Oregon")) %>% arrange(Cost) %>% group_by(State_Name, Year) %>% summarise(Cost = mean(Cost))farWestStatesHealthCare2014 %>% ggplot(aes( x = reorder(State_Name, Cost), y = Cost, fill = reorder(State_Name, Cost) )) + geom_col( aes( x = reorder(State_Name, Cost), y = Cost, fill = reorder(State_Name, Cost) ), color = "grey", show.legend = FALSE ) + geom_col( data = oregonHealthCare2014, aes( x = reorder(State_Name, Cost), y = Cost, fill = reorder(State_Name, Cost) ), color = "black", fill = "#154733", size = 1.5, show.legend = FALSE ) + geom_label(aes(label = print(paste0("$", round(Cost / 1000, 2), "M"))), fill = "white", size = 3 ) + geom_label( data = oregonHealthCare2014, aes(label = print(paste0("$", round(Cost / 1000, 2), "M"))), fill = "#FEE123", size = 3 ) + labs( x = "State", y = "Cost (USD)", title = "How Oregon Compares To The Far West States For Healthcare Costs" ) + scale_fill_manual(values = c("#fff5f0", "#fee0d2", "#fcbba1", "#fc9272", "#fb6a4a", "#ef3b2c")) + scale_y_continuous( labels = label_number(prefix = "$", suffix = "M", scale = 1e-3, big.mark = ","), breaks = c(10000, 20000, 30000, 40000, 50000) ) + theme_minimal() + theme(title = element_text(size = 10, face = "bold"))```### Inappropriate Model: Linear Fit Inadequate for the DataAt first glance, the model may seem impressive with an adjusted R-squared value of 0.8572. However, this is deceptive. It's crucial to note that this model is highly inaccurate and strongly discouraged. The analysis reveals no correlation between Cost and Region_Name per Year, a finding consistent with the filtered dataset covering the years 1980 to 2014.The residual plots provide clear evidence against a linear fit. The Residuals vs Fitted plot indicates a clear quadratic relationship rather than a linear one. The Q-Q plot deviates from linearity, exhibiting multiple curves along the fitted line. Additionally, the scale-location plot highlights that this model is fundamentally unsuitable for the data.It is evident that a linear fit is not the appropriate choice for accurately modeling this dataset.```{r message=FALSE, warning=FALSE, echo = FALSE}# Question: Are there no significant differences in spending for each region?regionHealthCareSince2005 <- ds_years %>% filter((Year >= 1980 & Year <= 2005) & Region_Name != "United States") %>% arrange(Cost) %>% group_by(Region_Name, Year) %>% summarise(Cost = mean(Cost))regionHealthCareSince2005 %>% ggplot(aes(x = Region_Name, y = Cost, color = Region_Name)) + geom_boxplot(color = "#b32134", size = 0.5, bins = 40) + labs( x = "Year", y = "Cost (USD)", title = "U.S. Healthcare Spending Distribution Per Region Since 2005" ) + scale_y_continuous(labels = label_number(prefix = "$", suffix = "M", scale = 1e-3, big.mark = ",")) + theme_classic() + theme( axis.text.x = element_text(angle = 90), strip.text = element_text(size = 6.5, face = "bold"), title = element_text(size = 10, face = "bold") )regionHealthCareSince2014 <- ds_years %>% filter(Region_Name != "United States") %>% arrange(Cost) %>% group_by(Region_Name, Year) %>% summarise(Cost = mean(Cost))regionHealthCareSince2014 %>% ggplot(aes(x = Region_Name, y = Cost, color = Region_Name)) + geom_boxplot(color = "#b32134", size = 0.5, bins = 40) + labs( x = "Year", y = "Cost (USD)", title = "U.S. Healthcare Spending Distribution Per Region Since 2014" ) + scale_y_continuous(labels = label_number(prefix = "$", suffix = "M", scale = 1e-3, big.mark = ",")) + theme_classic() + theme( axis.text.x = element_text(angle = 90), strip.text = element_text(size = 6.5, face = "bold"), title = element_text(size = 10, face = "bold") )model <- lm(data = regionHealthCareSince2005, Cost ~ Region_Name + Year)plot(model)test <- data.frame(Region_Name = "Far West", Year = 2006)prediction <- predict(model, test)predictionsummary(model)summary.aov(model)```### Significant Differences in Means among RegionsMy objective was to investigate whether there were significant differences in the means of each region's spending over the years. To start, I utilized the LeveneTest to examine the importance of variance (i.e., the spread of spending) across regions. Both tests yielded remarkably small p-values, indicating that three regions had substantially different variances compared to the others.Building on this, I employed the TukeyHsd test to confirm if these differing variances were reflected in the means. As anticipated from the LeveneTest results, the means of these regions indeed exhibited significant differences. Notably, New England, Plains, and Rocky Mountains had considerably lower average spending. However, despite their comparatively lower spending, these regions still followed the overall growth trend, with an increase of 18% since 2005.```{r message=FALSE, warning=FALSE, echo = FALSE}expensiveRegions2005 <- regionHealthCareSince2005 %>% filter(Region_Name != c("New England", "Plains", "Rocky Mountains"))expensiveRegions2014 <- regionHealthCareSince2014 %>% filter(Region_Name != c("New England", "Plains", "Rocky Mountains"))cheapRegions2005 <- regionHealthCareSince2005 %>% filter(Region_Name == c("New England", "Plains", "Rocky Mountains"))cheapRegions2014 <- regionHealthCareSince2014 %>% filter(Region_Name == c("New England", "Plains", "Rocky Mountains"))exp2005 <- round(mean(expensiveRegions2005$Cost), 2)exp2014 <- round(mean(expensiveRegions2014$Cost), 2)chp2005 <- round(mean(cheapRegions2005$Cost), 2)chp2014 <- round(mean(cheapRegions2014$Cost), 2)print(paste0("The Average Spending In The Expensive Regions since 2005 = $", exp2005))print(paste0("The Average Spending In The Expensive Regions since 2014= $", exp2014))print(paste0("Difference: +$", exp2014 - exp2005, " | Percentage Increase: +", round(((exp2014 - exp2005) / (exp2014 + exp2005)) * 100, 2), "%"))print(paste0("The Average Spending In The Cheap Regions since 2005 = $", chp2005))print(paste0("The Average Spending In The Cheap Regions since 2014= $", chp2014))print(paste0("Difference: +$", chp2014 - chp2005, " | Percentage Increase: ", round(((chp2014 - chp2005) / (chp2014 + chp2005)) * 100, 2), "%"))# Levene’s testleveneTest(Cost ~ Region_Name, data = regionHealthCareSince2005, center = mean)# Brown-Forsythe testleveneTest(Cost ~ Region_Name, data = regionHealthCareSince2005, center = median)# Levene’s testleveneTest(Cost ~ Region_Name, data = regionHealthCareSince2014, center = mean)# Brown-Forsythe testleveneTest(Cost ~ Region_Name, data = regionHealthCareSince2014, center = median)# Given both tests yield an extremely small p-value, it is clear that there are 3 regions that have a completely different variance than the other regions.# one way anova modelmodel <- aov(Cost ~ Region_Name, data = regionHealthCareSince2005)summary(model)# Let's do a TukeyHSD test to confirm this.TukeyHSD(model, conf.level = .95)plot(TukeyHSD(model, conf.level = .95), las = 2)# New England, Plains and Rocky Mountains spend much lower on average. Despite that, they are following the trend of growth with an 18% since 2005.```## ResultsThe cost of healthcare in the United States has increased more than fivefold between 1980 and 2014. Across regions, there is a consistent upward trend in healthcare spending with no clear indications of a decrease. Although some regions are less expensive than others, their growth rates align with the national average. Personal health care spending, which averaged around \$10,000 in 1980, has significantly risen to nearly \$80,000 in 2014.As of 2014, the Mideast, Great Lakes, and Far West regions rank as the top three most expensive regions, while the Rocky Mountains, New England, and Plains regions are the least expensive.Within the Far West region, Oregon stands out as the third most expensive state.## ConclusionThe United States continues to experience escalating healthcare expenditures, raising concerns about the affordability of personal health care. The substantial increase of approximately \$70,000 over a span of 35 years far exceeds inflation expectations. It would have been beneficial to have inflation-adjusted values in the dataset, allowing for a more comprehensive analysis and deeper insights.Further exploration can be done to investigate potential statistical significance between individual states and their spending patterns. This avenue remains open for future researchers to delve into for a more in-depth understanding of healthcare expenditure variations.## Data References```{r, echo=FALSE}knitr::write_bib(names(sessionInfo()$otherPkgs), file="bibliography.bib")```