Code
library(shiny)
library(tidyverse)
library(readr)
library(stringr)
library(plotly)
library(DT)
options(scipen = 999)
Brian Cervantes Alvarez
April 21, 2023
June 16, 2025
I built a Shiny dashboard to analyze Amazon products in India by integrating multiple datasets, performing extensive data wrangling, and applying advanced CSS theming to enhance design and efficiency. I developed interactive visualizations using Plotly and DT to display average product ratings and review counts across categories, facilitating easy exploration and comparison. My work demonstrates strong skills in data processing, dynamic UI development, and effective dashboard design for clear, insightful data analysis.
This project focuses on the development of a Shiny application to analyze Amazon products in India. Using a dataset sourced from Kaggle, the application aims to create an informative dashboard showcasing categories with the highest average ratings and reviews. Despite encountering challenges with product categorization, the project prioritizes efficiency and design improvements.
The objective of this project is to create a Shiny application that explores the Amazon products dataset from India. The dataset, obtained from Kaggle, presented challenges during development, particularly regarding product categories. However, the project’s primary focus was on creating a concise and informative dashboard displaying categories with the highest average ratings and reviews.
Compared to previous Shiny app development experiences, this project demonstrated improved efficiency, taking significantly less time to complete. Furthermore, advanced CSS theming was implemented to enhance the overall design of the application. While additional features and visualizations could be incorporated, the decision was made to leave them for future exploration.
What is the average rating for each category and subcategory?
What is the average review for each category and subcategory?
This project served as a rewarding experience, providing opportunities to enhance data analysis and Shiny app development skills. The Shiny application developed enables users to analyze Amazon products in India and offers insights into categories with the highest average ratings and reviews. Despite encountering challenges related to product categorization, the project prioritized efficiency and introduced improvements in design. Future exploration of additional features and visualizations remains open for further development and enhancement.
# ORIGINAL DATA WRANGLING
datasets <- as.data.frame(list.files(path = "~/Documents/ShinyApps/AmazonProducts/AmazonProductApp", pattern = "csv"))
colnames(datasets) <- "Datasets"
# Combine all the datasets
for (i in length(nrow(datasets))){
combinedDs <- read_csv(datasets[[i]])
}
amazonProducts <- combinedDs %>%
mutate(Name = name,
MainCategory = factor(str_to_title((sort(main_category)))),
SubCategory = factor(sort(sub_category)),
ProductImage = image,
ProductRating = as.numeric(ratings),
NumberOfRatings = as.numeric(gsub("\\,","",no_of_ratings)),
DiscountPrice = round(as.numeric(gsub("[\\₹,]", "", discount_price)) / 81.85, 2), # convert from Rupee to USD
Price = round(as.numeric(gsub("[\\₹,]", "", actual_price)) / 81.85, 2), # convert from Rupee to USD
ProductLink = link) %>%
select(-c(name,
main_category,
sub_category,
image,
ratings,
no_of_ratings,
discount_price,
actual_price,
link)) %>%
drop_na() %>%
filter(!str_detect(SubCategory, "^All "))
# amazonProducts %>%
# write_csv("amazonProducts.csv")
products <- products %>% mutate(DiscountPrice = round(DiscountPrice82.04,2), Price = round(Price82.04,2))
#For Plot 1
ratings <- products %>% select(-c(ProductImage, Name, ProductLink)) %>% group_by(MainCategory,SubCategory) %>% summarise(AverageRating = mean(ProductRating)) %>% ungroup()
reviews <- products %>% select(-c(ProductImage, Name, ProductLink)) %>% group_by(MainCategory,SubCategory) %>% summarise(AverageReview = mean(NumberOfRatings)) %>% ungroup()
#For Plot 2
top10Products <- products %>% filter(ProductRating > 4.5, NumberOfRatings > 50) %>% group_by(SubCategory) %>% arrange(desc(ProductRating)) %>% slice(1:10) %>% select(-c(ProductImage, Name, ProductLink))
#unique(products$MainCategory)
# Define UI for application
ui <- fluidPage(
#Background CSS
tags$head(tags$style(HTML('
@import url(https://fonts.googleapis.com/css?family=Montserrat&display=swap);
body {
font-family: Montserrat, sans-serif;
background-color: #FF9900;
}
.dataTables_wrapper {
background-color: #fff;
}
.sidebar {
background-color: #fff;
width: 3/12;
height: 2/12;
}
.nav-tabs > li > a {
color: black;
background-color: #00a8e1;
border-color: #00a8e1;
}'))),
# Application title
titlePanel("Amazon Inc. Product Dashboard (EN.)"),
# Sidebar
sidebarLayout(
sidebarPanel(
selectInput("MainCategoryChoice",
label = h3("Select Category:"),
choices = unique(products$MainCategory),
selected = "Accessories"),
uiOutput("SubCategoryChoice")
),
# Tabs
mainPanel(
tabsetPanel(
tabPanel("Plot",
plotlyOutput("RatingsPlot"),
plotlyOutput("ReviewsPlot")),
tabPanel("Data", dataTableOutput("myDataTable"))
)
)
)
)
# Define server logic required to draw Dashboard
server <- function(input, output) {
# Subcategory choices
output$SubCategoryChoice <- renderUI({
subcategories <- unique(products$SubCategory[products$MainCategory == input$MainCategoryChoice])
checkboxGroupInput("SubCategoryChoice",
label = h3("Select Subcategories:"),
choices = subcategories,
selected = subcategories)
})
# Plot 1: Product Rating
output$RatingsPlot <- renderPlotly({
ratings %>%
filter(MainCategory == input$MainCategoryChoice,
SubCategory %in% input$SubCategoryChoice) %>%
mutate(SubCategory_ordered = factor(SubCategory,
levels = unique(SubCategory[order(AverageRating)]))) %>%
plot_ly(x = ~SubCategory_ordered,
y = ~round(AverageRating,2),
type = 'bar',
marker = list(color = ~pal1[SubCategory_ordered])) %>%
add_annotations(x = ~SubCategory_ordered,
y = ~round(AverageRating,2),
text = ~paste0(round(AverageRating,2)),
font = list(color = 'black',
size = 10),
showarrow = FALSE,
yshift = 5) %>%
layout(title = paste0("Average Product Rating For ",input$MainCategoryChoice),
xaxis = list(title = "", tickangle = 45),
yaxis = list(title = ""),
showlegend = FALSE,
margin = list(t = 50,
l = 50,
r = 50,
b = 50))
})
# Plot 2: Product Reviews
output$ReviewsPlot <- renderPlotly({
reviews %>%
filter(MainCategory == input$MainCategoryChoice,
SubCategory %in% input$SubCategoryChoice) %>%
mutate(SubCategory_ordered = factor(SubCategory,
levels = unique(SubCategory[order(AverageReview)]))) %>%
plot_ly(x = ~SubCategory_ordered,
y = ~round(AverageReview),
type = 'bar',
marker = list(color = ~pal2[SubCategory_ordered])) %>%
add_annotations(x = ~SubCategory_ordered,
y = ~round(AverageReview),
text = ~paste0(round(AverageReview)),
font = list(color = 'black',
size = 10),
showarrow = FALSE,
yshift = 5) %>%
layout(title = paste0("Average Number of Reviews For ",input$MainCategoryChoice),
xaxis = list(title = "", tickangle = 45),
yaxis = list(title = ""),
showlegend = FALSE,
margin = list(t = 50,
l = 50,
r =50,
b = 50))
})
output$myDataTable <- DT::renderDataTable({
products %>%
filter(MainCategory == input$MainCategoryChoice,
SubCategory %in% input$SubCategoryChoice) %>%
mutate(ProductImage = sprintf('<img src="%s" width="75px"/>', ProductImage)) %>%
DT::datatable(., escape = FALSE, options = list(
pageLength = 10,
lengthMenu = c(5, 10, 25),
scrollY = "600px",
scrollX = TRUE
)) %>%
DT::formatStyle(columns = colnames(products),
backgroundColor = styleEqual(c("green", "white"), c("rgb(51, 102, 0)", "rgb(255, 255, 255)")))
})
}
# Run the application
shinyApp(ui = ui, server = server)
---
title: "Building A Quick Dashboard For Amazon Products (EN.)"
author: "Brian Cervantes Alvarez"
date: "04-21-2023"
date-modified: today
image: /assets/images/AmazonLogo.jpeg
description: "Explore Amazon products in India with our Shiny app. Discover categories with the highest ratings and reviews. Efficient, informative, and visually appealing."
bibliography: "bibliography.bib"
nocite: |
@*
format:
html:
code-tools: true
code-fold: true
toc: true
toc-location: right
html-math-method: katex
page-layout: article
execute:
eval: false
message: false
warning: false
categories: [R, Shiny, CSS, Data Tables, Data Visualization, Dashboard]
ai-summary:
banner-title: "Yapper Labs | AI Summary"
model-title: "Model: ChatGPT o3-mini-high"
model-img: "/assets/images/OpenAI-white-monoblossom.svg"
summary: "I built a Shiny dashboard to analyze Amazon products in India by integrating multiple datasets, performing extensive data wrangling, and applying advanced CSS theming to enhance design and efficiency. I developed interactive visualizations using Plotly and DT to display average product ratings and review counts across categories, facilitating easy exploration and comparison. My work demonstrates strong skills in data processing, dynamic UI development, and effective dashboard design for clear, insightful data analysis."
---
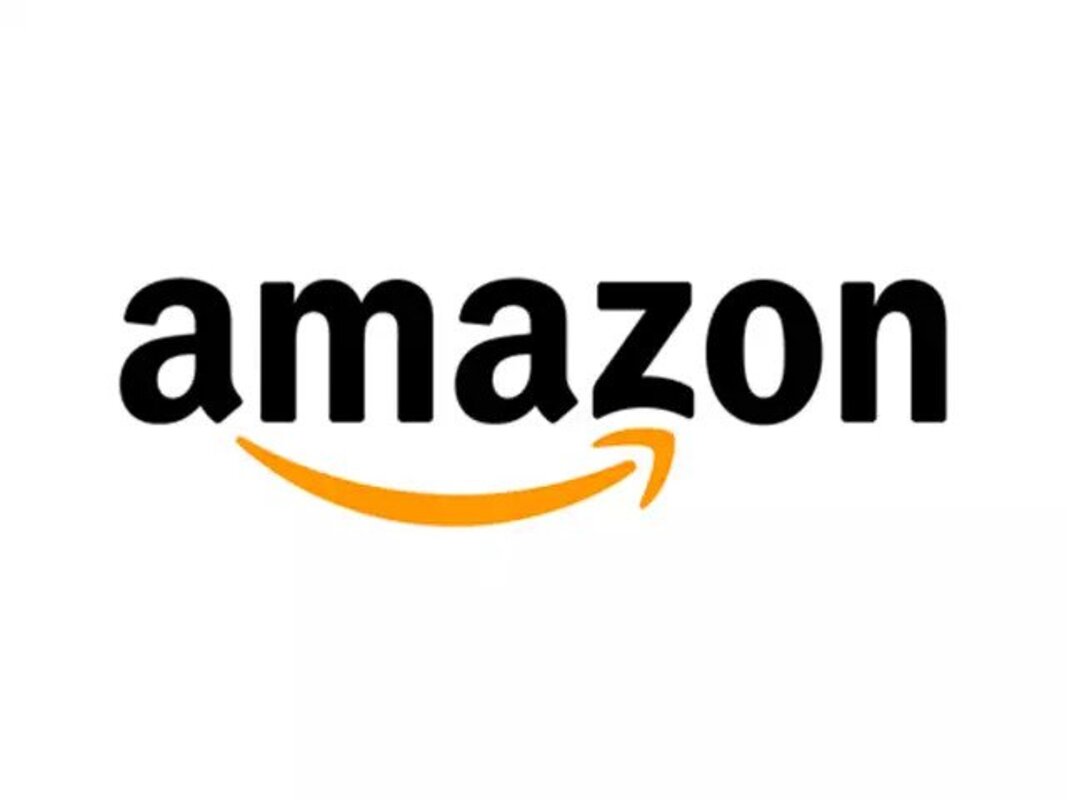
## Abstract
This project focuses on the development of a Shiny application to analyze Amazon products in India. Using a dataset sourced from Kaggle, the application aims to create an informative dashboard showcasing categories with the highest average ratings and reviews. Despite encountering challenges with product categorization, the project prioritizes efficiency and design improvements.
## Introduction
The objective of this project is to create a Shiny application that explores the Amazon products dataset from India. The dataset, obtained from Kaggle, presented challenges during development, particularly regarding product categories. However, the project's primary focus was on creating a concise and informative dashboard displaying categories with the highest average ratings and reviews.
Compared to previous Shiny app development experiences, this project demonstrated improved efficiency, taking significantly less time to complete. Furthermore, advanced CSS theming was implemented to enhance the overall design of the application. While additional features and visualizations could be incorporated, the decision was made to leave them for future exploration.
## Questions
- What is the average **rating** for each category and subcategory?
- What is the average **review** for each category and subcategory?
## Further Improvement Questions
- Is there a correlation between the number of ratings and the product rating?
- What is the average discount percentage for each main category and subcategory?
- What is the price range for each main category and subcategory?
- Which products have the highest ratings and how do they compare in terms of price and number of ratings?
## Conclusion
This project served as a rewarding experience, providing opportunities to enhance data analysis and Shiny app development skills. The Shiny application developed enables users to analyze Amazon products in India and offers insights into categories with the highest average ratings and reviews. Despite encountering challenges related to product categorization, the project prioritized efficiency and introduced improvements in design. Future exploration of additional features and visualizations remains open for further development and enhancement.
::: column-screen
<iframe src="https://bcervantesalvarez.shinyapps.io/AmazonProductApp/" title="UKAccidentTimeSeries" width="100%" height="800">
</iframe>
:::
[Fullscreen](https://bcervantesalvarez.shinyapps.io/AmazonProductApp/)
# Appendix
## Load Libraries
```{r, eval=TRUE,output=FALSE}
library(shiny)
library(tidyverse)
library(readr)
library(stringr)
library(plotly)
library(DT)
options(scipen = 999)
```
## Part 1: Data Wrangling Multiple Datasets
```{r}
#| eval: false
# ORIGINAL DATA WRANGLING
datasets <- as.data.frame(list.files(path = "~/Documents/ShinyApps/AmazonProducts/AmazonProductApp", pattern = "csv"))
colnames(datasets) <- "Datasets"
# Combine all the datasets
for (i in length(nrow(datasets))){
combinedDs <- read_csv(datasets[[i]])
}
amazonProducts <- combinedDs %>%
mutate(Name = name,
MainCategory = factor(str_to_title((sort(main_category)))),
SubCategory = factor(sort(sub_category)),
ProductImage = image,
ProductRating = as.numeric(ratings),
NumberOfRatings = as.numeric(gsub("\\,","",no_of_ratings)),
DiscountPrice = round(as.numeric(gsub("[\\₹,]", "", discount_price)) / 81.85, 2), # convert from Rupee to USD
Price = round(as.numeric(gsub("[\\₹,]", "", actual_price)) / 81.85, 2), # convert from Rupee to USD
ProductLink = link) %>%
select(-c(name,
main_category,
sub_category,
image,
ratings,
no_of_ratings,
discount_price,
actual_price,
link)) %>%
drop_na() %>%
filter(!str_detect(SubCategory, "^All "))
# amazonProducts %>%
# write_csv("amazonProducts.csv")
```
### Reload Data
```{r}
products <- read_csv("../../../Assets/Datasets/amazonProducts.csv")
```
## Part 2: Data Wrangling For Visualization
```{r}
products <- products %>% mutate(DiscountPrice = round(DiscountPrice82.04,2), Price = round(Price82.04,2))
#For Plot 1
ratings <- products %>% select(-c(ProductImage, Name, ProductLink)) %>% group_by(MainCategory,SubCategory) %>% summarise(AverageRating = mean(ProductRating)) %>% ungroup()
reviews <- products %>% select(-c(ProductImage, Name, ProductLink)) %>% group_by(MainCategory,SubCategory) %>% summarise(AverageReview = mean(NumberOfRatings)) %>% ungroup()
#For Plot 2
top10Products <- products %>% filter(ProductRating > 4.5, NumberOfRatings > 50) %>% group_by(SubCategory) %>% arrange(desc(ProductRating)) %>% slice(1:10) %>% select(-c(ProductImage, Name, ProductLink))
#unique(products$MainCategory)
```
### Color Theming
```{r}
#Plot 1
num_colors <- 21
colors <- c("#f2f2f2", "#ff9900")
pal1 <- colorRampPalette(colors)(num_colors)
print(pal1)
#Plot 2
num_colors <- 21
colors <- colors <- c("#f2f2f2","#00a8e1")
pal2 <- colorRampPalette(colors)(num_colors)
```
## Part 1: Shiny UI
```{r}
# Define UI for application
ui <- fluidPage(
#Background CSS
tags$head(tags$style(HTML('
@import url(https://fonts.googleapis.com/css?family=Montserrat&display=swap);
body {
font-family: Montserrat, sans-serif;
background-color: #FF9900;
}
.dataTables_wrapper {
background-color: #fff;
}
.sidebar {
background-color: #fff;
width: 3/12;
height: 2/12;
}
.nav-tabs > li > a {
color: black;
background-color: #00a8e1;
border-color: #00a8e1;
}'))),
# Application title
titlePanel("Amazon Inc. Product Dashboard (EN.)"),
# Sidebar
sidebarLayout(
sidebarPanel(
selectInput("MainCategoryChoice",
label = h3("Select Category:"),
choices = unique(products$MainCategory),
selected = "Accessories"),
uiOutput("SubCategoryChoice")
),
# Tabs
mainPanel(
tabsetPanel(
tabPanel("Plot",
plotlyOutput("RatingsPlot"),
plotlyOutput("ReviewsPlot")),
tabPanel("Data", dataTableOutput("myDataTable"))
)
)
)
)
```
## Part 2: Shiny Server
```{r}
# Define server logic required to draw Dashboard
server <- function(input, output) {
# Subcategory choices
output$SubCategoryChoice <- renderUI({
subcategories <- unique(products$SubCategory[products$MainCategory == input$MainCategoryChoice])
checkboxGroupInput("SubCategoryChoice",
label = h3("Select Subcategories:"),
choices = subcategories,
selected = subcategories)
})
# Plot 1: Product Rating
output$RatingsPlot <- renderPlotly({
ratings %>%
filter(MainCategory == input$MainCategoryChoice,
SubCategory %in% input$SubCategoryChoice) %>%
mutate(SubCategory_ordered = factor(SubCategory,
levels = unique(SubCategory[order(AverageRating)]))) %>%
plot_ly(x = ~SubCategory_ordered,
y = ~round(AverageRating,2),
type = 'bar',
marker = list(color = ~pal1[SubCategory_ordered])) %>%
add_annotations(x = ~SubCategory_ordered,
y = ~round(AverageRating,2),
text = ~paste0(round(AverageRating,2)),
font = list(color = 'black',
size = 10),
showarrow = FALSE,
yshift = 5) %>%
layout(title = paste0("Average Product Rating For ",input$MainCategoryChoice),
xaxis = list(title = "", tickangle = 45),
yaxis = list(title = ""),
showlegend = FALSE,
margin = list(t = 50,
l = 50,
r = 50,
b = 50))
})
# Plot 2: Product Reviews
output$ReviewsPlot <- renderPlotly({
reviews %>%
filter(MainCategory == input$MainCategoryChoice,
SubCategory %in% input$SubCategoryChoice) %>%
mutate(SubCategory_ordered = factor(SubCategory,
levels = unique(SubCategory[order(AverageReview)]))) %>%
plot_ly(x = ~SubCategory_ordered,
y = ~round(AverageReview),
type = 'bar',
marker = list(color = ~pal2[SubCategory_ordered])) %>%
add_annotations(x = ~SubCategory_ordered,
y = ~round(AverageReview),
text = ~paste0(round(AverageReview)),
font = list(color = 'black',
size = 10),
showarrow = FALSE,
yshift = 5) %>%
layout(title = paste0("Average Number of Reviews For ",input$MainCategoryChoice),
xaxis = list(title = "", tickangle = 45),
yaxis = list(title = ""),
showlegend = FALSE,
margin = list(t = 50,
l = 50,
r =50,
b = 50))
})
output$myDataTable <- DT::renderDataTable({
products %>%
filter(MainCategory == input$MainCategoryChoice,
SubCategory %in% input$SubCategoryChoice) %>%
mutate(ProductImage = sprintf('<img src="%s" width="75px"/>', ProductImage)) %>%
DT::datatable(., escape = FALSE, options = list(
pageLength = 10,
lengthMenu = c(5, 10, 25),
scrollY = "600px",
scrollX = TRUE
)) %>%
DT::formatStyle(columns = colnames(products),
backgroundColor = styleEqual(c("green", "white"), c("rgb(51, 102, 0)", "rgb(255, 255, 255)")))
})
}
# Run the application
shinyApp(ui = ui, server = server)
```